
Ready to make smarter marketing decisions? Focus on profitability!
The idea of making marketing decisions that are primarily based on historical data sounds great, doesn’t it? I mean, just a couple of decades ago, such a capability would have been a distant (and ridiculously costly) dream for marketing teams. There were also obstacles, such as lack of tools and understanding that got in the way.
Over time, the practice of using historical data to make marketing decisions came underway, and became heralded for fostering a whole new environment for growth. It became clear that historical data is essential for discovering cause-and-effect patterns. It can help to identify and predict trends, as well as the enhancement of customer relations, pinpointing market needs, and accelerating business growth and profitability.
However, what people tend to talk less about are the difficulties that are associated with making quick decisions that are solely based on historical and limited real-time data (based on the performance of the campaign at-hand,) plus the fact that predictive analytics helps marketers make smarter decisions.
Before I get into the value that predictive analytics gives marketing teams in terms of profitability, I’ll start off by talking about the pressure points.
Difference in difficulties between industries
Offhand, it might seem like a sweet deal to be able to quickly make decisions based on historical, and any degree of real-time data. But it all works a little differently for different types of businesses.
{{banner}}
In the case of D2C subscription brands, for instance, it’s relatively easy to get an understanding of where subscribers stand, because conversions happen quickly, and first-month revenues are seen quickly. But there are some other crucial details that end up falling through the cracks, particularly that of churn and loyalty. With historical and limited real-time data, you won’t be able to determine how many of them are likely to become loyal subscribers, or even the future value of the cohort. After all, a decent percentage of them will upgrade their subscription packages, or capitalize on add-ons, but none of this can be manually predicted based on limited insights.
Consumer subscription brands, such as Noom, Duolingo, Grammarly, Calm, Spotify, Future, and Flo have managed to stand the test of time for many reasons. In addition to their obsession with efficiency, these brands also have an “alignment between product strategy and acquisition strategy,” and a “singular focus to build a magical, sticky product through rapid iteration and endless optimization.”
When it comes to SaaS B2B brands, which are obviously structured very differently from D2C subscription brands, it’s a whole different set of obstacles. Conversions take a little longer to pan out due to entirely different customer journeys and longer funnels. This is especially the case for brands that offer free trials, or freemium packages, because there are hardly any real conversions early on. There are only maybe soft conversions at best, such as basic registration, the start of the free trial, and brand/product engagement.
As the saying goes, haste makes waste. That definitely applies towards growth teams that hastily make marketing decisions based on limited real-time data and historical averages, because these decisions are stuck in the past. They don’t factor, or reflect future performance.
Healthy unit economics leads to profitability
Historical data itself isn’t all-encompassing, and doesn’t help in terms of predictive intel on the future success of campaigns.
The greatest challenge is the lack of access to meaningful data with deeper marketing value. The timeliness and accessibility of data is hardly the issue at all. The thing is—it doesn’t provide a way for growth teams to fuel and scale user acquisition efforts while maintaining healthy unit economics, such as CPA, CAC, and ROAS. Healthy unit economics are a must for data-driven companies that are looking to build profitability over growth at any cost—and ROI needs to be positive. After all, a growth strategy that doesn’t use profitability as a starting point only ends up leading to lower levels of growth, thereby lackluster profitability.

Many useful insights end up going down the drain, and this is where predictive analytics comes in.
Predictive analytics, as the name denotes, forecasts what is likely to happen based on patterns in collected data, and presents multiple use cases.
In general, there are numerous use cases for predictive analytics simply because it uses historical data to predict trends and behavior, thereby enabling companies to build better products and provide better service.
When it comes to growth marketing, especially for subscription brands, predictive analytics is used for segmenting, targeting, acquiring and retaining customers. It is also used to determine what kinds of ads to show, and which would be most effective to show prospective customers. It gets even deeper than that for growth teams, as it helps set the groundwork for long-term profitability and scalability. Without predictive analytics, determining the long-term value of a cohort can take months for subscription brands, and is far quicker with the backing of a predictive marketing solution.
What makes predictive analytics and insights valuable for marketing and growth teams
So why would marketing and growth teams even be interested in predictive analytics and insights in the first place? Well… imagine being able to know your users' value in the first six months, today. Imagine being able to measure a campaign or ad group's performance 12 months ahead, after the first few days. It’s like deep insider knowledge that gives you the upper hand.
Allow me to explain. In the table below, you’ll see two campaigns are being compared using real-time data—basically showing how the campaigns are performing to date:
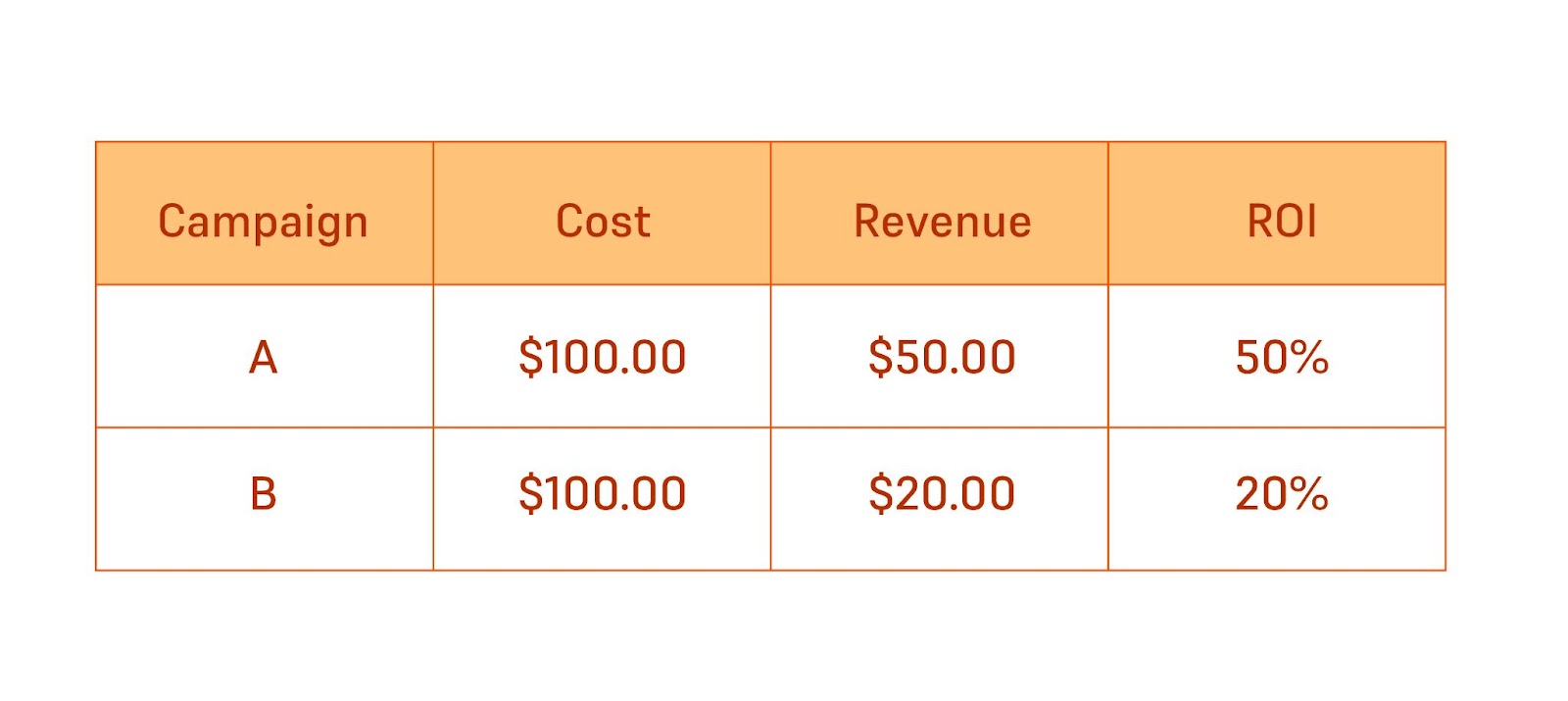
Which campaign would you keep?
Which campaign would you kill?
Looking at Predicted ROI will surely change the course of your decision.

As you know, marketing teams had a lot of surprises thrown at them in the past year, and they weren’t the fun kind. These surprises included limitations of ad networks’ native capabilities, new privacy policies, and regulations, and limited availability of supporting services and technologies.
These collectively placed your team in the position to use outdated data to support quick and early evaluation of trends and performance of their user acquisition campaigns. As a result, you’re not maximizing the potential of your growth campaigns, which in turn negatively affects your brand’s long-term profitability.
This is where predictive analytics steps in, essentially serving as the light at the end of the tunnel.
By turning to predictive analytics, you essentially arm your growth team with the LTV-optimized insights they can use to understand how different campaigns will perform in the future, and act based on that intel. They are given all the context and data points needed to ensure the success of each campaign. Predictive analytics is kind of like a crystal ball for growth marketing campaigns, and in the case of our own AI and ML-powered solution, we call it Predictive Insights.
Predictive analytics in general helps bridge the gaps that have long been experienced by growth teams, by providing them with the information they need in order to make informed, time-sensitive decisions on their campaigns. I’m sure you can imagine what a time saver that collectively becomes, when managing multiple marketing channels and campaigns, by using AI to unlock the ability to optimize on a daily basis, based on real-time.
The LTV-optimization element is what makes this particularly meaningful, and valuable for growth teams. Your marketing team has much to gain with the ability to leverage zero- and first-party data to predict the LTV and ROAS on a single campaign or cohort level, shortly after launching a new campaign, or even changing an existing one.
Taking core elements of historical data, and making it better
To loop back to making decisions solely based on historical data, it’s certainly great for brands that are more about quick wins. But if your subscription brand is looking to determine what can happen in the future, based on what has happened in the past, LTV-optimized predictive marketing is the way to go.
This will enable your team to make better informed decisions with each campaign. They can also use predictive analytics to accurately evaluate ad spend impact and effectiveness at any level of granularity, including the detection of cohort trends, gauging projected performance of creative and copy, and so much more.
It’s safe to say that with the backing of a tool such as predictive insights, marketing teams become equipped to make informed decisions with clarity and confidence. Gone are the days of gambling with user acquisition campaigns by acting on limited data, and counting on luck, because decisions that are based on predictive analytics are made with the assurance that it is the best route to take for long-term impact and profitability.
Subscribe for more
Read expert stories, interviews, reports, insights and tips for profitable growth.