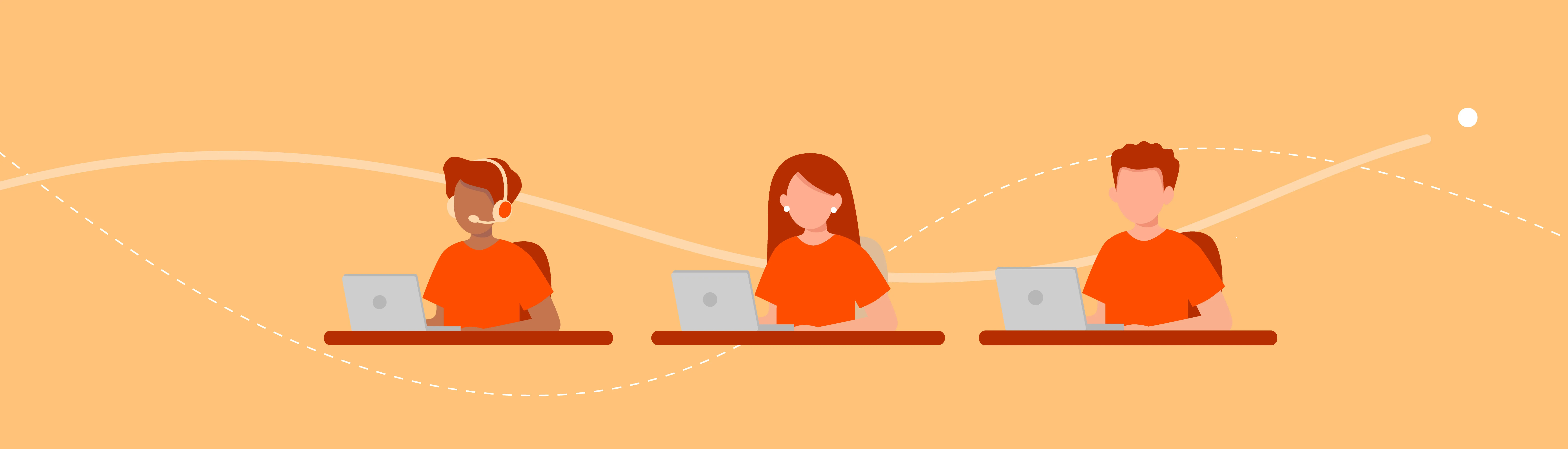
Do it in-house: Use LTV projections as part of the value-based acquisition process
Welcome to the second installment of our “Do it in-house” series!
In the first “Do it in-house” post, Transitioning from 1-CAC-fits-all, to the value modeling approach, we went over how subscription SaaS B2B companies can easily switch to the value modeling approach in their ad campaigns—in-house!
If you didn’t get a chance to read that post, I highly recommend it, because in addition to explaining the process, we went over the current state of B2B campaign optimization, and the formula to project CAC using past conversion data.
In continuation—this post builds on projected CAC and takes it to the next level, to project LTV and use that for evaluating and optimizing ad campaigns. And how you can do it in-house. 🙂
{{banner}}
The benefits of using projected LTV
Many companies these days use observable ROAS (returns observed early on, day1, or day 7, on ad platforms shortly after acquisition) for decision making. The result is typically extremely low ROAS with high CAC. Furthermore, these are based on an extremely low number of actual conversions, and are thus very statistically unstable. Considering the fact that most subscription brands offer a freemium usage funnel, or a free trial, which leads to most of the eventual conversions occurring outside the attribution/optimization window. On the flip side, using projected LTV allows making decisions based on projected ROAS, which is itself based on many more numerous, and more stable data points. This leads to a wide range of benefits that I’ll explain below.
It’s important for subscription SaaS B2B companies to understand that there’s so much more to projected LTV data than what meets the eye. Use cases for it include:
- The ability to look at long-term revenue and profitability
- Helps marketing teams make better informed, early-on keep/kill decisions
In order to achieve sustainable growth and profitability, it is crucial for SaaS businesses to make their marketing decisions based on LTV data. It is an important metric because of how much retention and engagement correlates with SaaS profitability, and the fact that LTV is such an effective predictive tool for future marketing decisions is a godsend.
Okay, now let’s get to the meat of things! Here’s the process for your team to take on LTV projections in-house!
How to take on value modeling in-house using LTV projections
As we stated in our previous post, the value modeling approach will allow your marketing team to make more informed marketing decisions that look beyond the first few days. It also helps your brand get more bang for its buck, as you will be able to pay for cohorts in accordance with their eventual LTV.
The following visualization demonstrates how to activate value modeling as an ongoing process:

Now here’s how you can get started on that, using LTV projections.
Be sure to keep note of the result after completing each formula, as you would need them to proceed with next steps.
You begin by calculating the value of a paying team on day 90 (D90 is an example here, use whatever future period you are interested in) using the following formula:

The second stage is to calculate the ratio between number of paying teams on day 90, to number of paying teams on day 1 (D1, again, is an example, choose D3 or D7 etc. if that’s when you want to take an action on marketing cohorts) as seen below:

For the final stage, using the two factors, you will be able to calculate the projected revenue, as seen below:

You can then easily translate this to the pLTV for a free team in your cohort (which are numerous and observable in the early days).

And the projected ROAS is then:

Read on for a deeper look into the method to the madness of the formula above.
Deeper explanation of projected revenue
The projected revenue from paying teams is the number of paying teams on day one multiplied by the ratio factor projecting the ratio of paying teams on day 90 (previously calculated on stage 2), multiplied by the projected value of a paying team on day 90 (previously calculated on stage 1).
Here’s an example of using projected revenue:
- XYZ is a SaaS B2B company
- Their CFO capped the CAC for paying teams at $600
- The marketing team ran a campaign with $20K media spend
- On it’s first day the campaign yields 15 paying teams and $1,500
Let’s assume that their historical data from 2021 shows:
- XYZ spent $10M on Google search
- The campaigns yielded 4,000 paying teams by day 1
- The campaigns yielded 10,000 paying teams, and 50,000 signed up teams by day 90
- XYZ generated $4.5M by day 90
Now let’s analyze the new campaign using projected revenue:

Based on the XYZ’s historical data, the projected ROAS of this campaign on day 90 is 84%.
✨✨Now the marketing team can make an informed decision regarding the campaign's performance.✨✨
Advanced projections using segmentation
As a hands-on growth expert, you know that there is volatility in the value of different teams. It is typically based on geo, channels, devices, company size and more.
Using one LTV projection will not reflect those differences. To the contrary, using a single LTV projection across the board will result in inaccurate decisions (you may kill campaigns of more profitable audiences, as they will be modeled as an average over all your audiences).
As such, the next stage of the process is to generate and use different projections for different segments, to enhance your marketing team's capability to make an accurate evaluation.
The following is an example of how the projected LTV can differ from geo to geo:
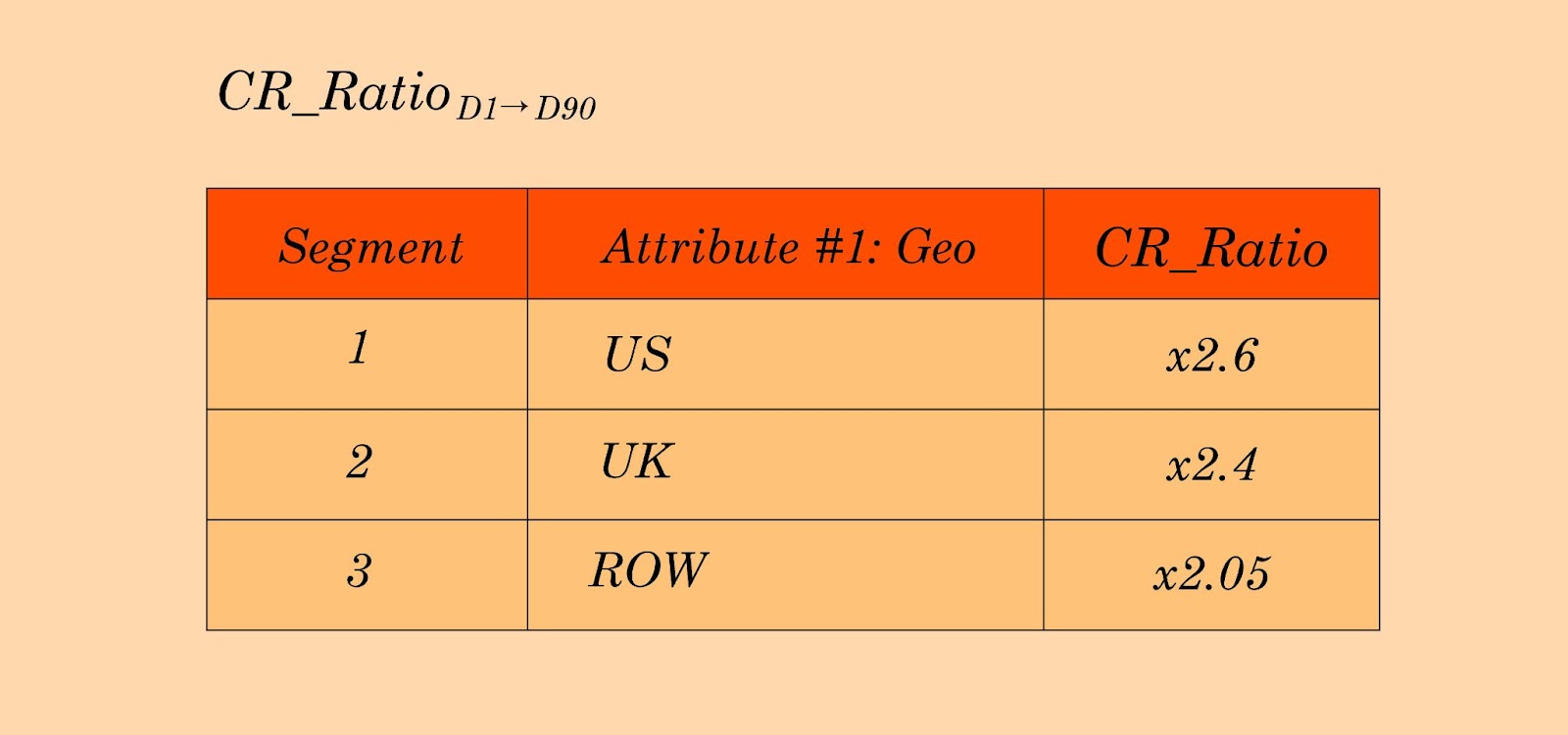
Where common practices fall short
At this point, you may be wondering what the point of all this is. If existing common practices are working, why should one consider changing their growth practices? It’s mainly because in the ever-changing landscape, common practices are falling short in a few areas:
Generalization
Common solutions are one-size-fit-most - and do not necessarily fit all types of businesses.
Maintenance
The value multipliers are rarely recalculated or adjusted - resulting in inaccuracy and sometimes even irrelevancy.
Testing/Visibility
There are no mechanisms in place to test the sensitivity and impact of value adjustments. That causes the stagnant value multipliers mentioned in the previous point, and company-wide hesitation to take responsibility on updating those “models”
Scale
The multiplier approach will break at 3+ dimensions, since the segments become too small, and the statistical significance starts to break.
Bear in mind that too many dimensions can create too small of an audience to properly calculate pLTV. I’ll show you what I mean.
The following visualization shows how it works well with one dimension:
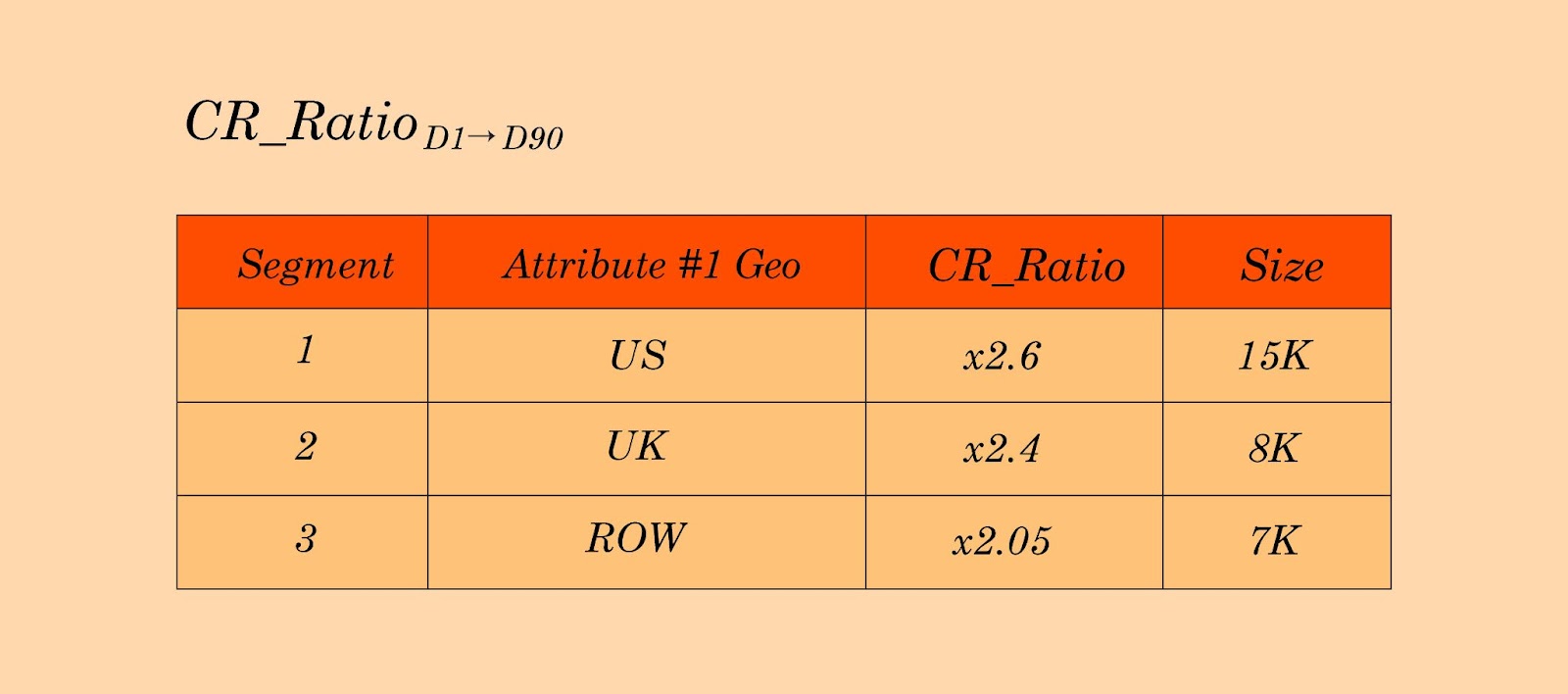
Interestingly enough, the audience size becomes reduced with two dimensions (if they have low cardinality, just 2-4 options), but still supports the projection:
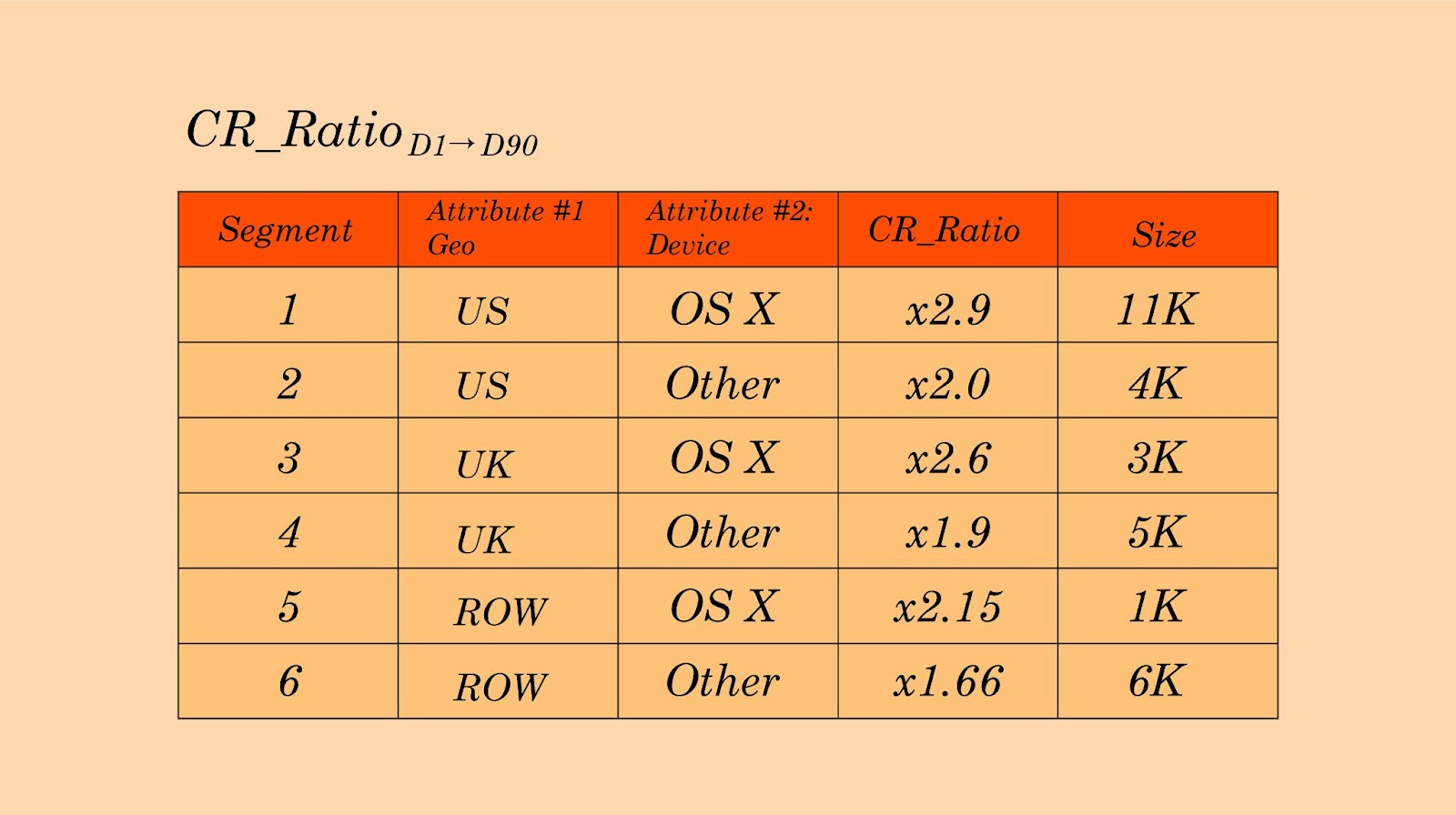
Now here’s where we’re entering the danger zone. With added dimensions, the audience size has become far too small:

While “lookup table” approach breaks down with narrower segmentation, a machine learning model would know play around with the correct segmentation parameters - for example, in the RestOfWorld (ROW) it can look into device and search term, while in the US it might not split on device, and just split on the search term.
This approach is infinitely scalable with other parameters, when you leverage machine learning, and this is where the true predictive power waits to be harnessed.
ML-based predictive bidding refers to the usage of predictive models that stem from LTV data, to specifically target the most valuable users that demonstrate high LTV.
Stay tuned for our next post, to find out more about predictive bidding, and how your team can do it in-house!
Subscribe for more
Read expert stories, interviews, reports, insights and tips for profitable growth.