
Consider this principle when analyzing data to attract the most valuable users
The Pareto Principle, which is also known as the 80/20 rule, is known for showing itself in many aspects of business development, regardless of the industry.
Interestingly enough, understanding and strategizing based on this principle can totally change the whole way you’re thinking about marketing. In all the best ways, of course. 🙂
Now I assure you that I’m no psychic, but I’m quite certain that a very small percentage of your users are responsible for the majority of your revenue. No need to freak out over my statement, that’s the case with most brands, regardless of the vertical. Especially for brands that are focused on loyalty.
But the conversation doesn’t end here with that interesting factoid. If anything, the conversation is only starting if you want to talk about how this applies to the amplification of your user acquisition efforts.
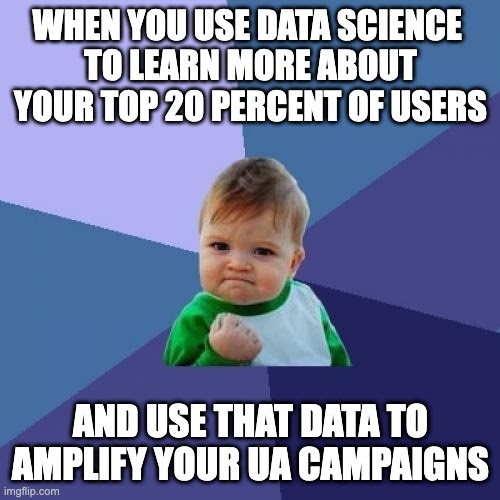
This is where quantity meets quality
You see, that small percentage of people that’s bringing in the revenue—they clearly have a high LTV. Your brand needs more of those! You also need to bear the flip-side in mind. While acquiring more of this audience is very important, it also means that a majority of the audience you’re bringing to your campaigns are not a high LTV audience.
You need to drill down into this thought process as a data scientist would, to make more sense of how to approach your UA campaigns. Acquiring more users with high LTV, like your ideal cohort, will significantly impact your revenue and profits.
By using LTV models and being able to identify new users that are to become those types of high LTV users, the added benefit to your brand is very significant. There is proof that focusing on LTV, and being able to focus on LTV from user acquisition can change the outcome of your campaigns, and what the return of your campaigns can be.
Find out more about the users with high LTV
In order to learn more about your users with high LTV, you need to first identify the users who have bought recently, frequently and spent the most.
From there, it is helpful to find out where they come from -- the traffic channels that they discovered, the ads on which they converted and the content with which they engaged. By doing so, you can optimize, enhance and promote that content.
You would also want to focus on the ad networks that most of your best users are coming from.
You can also perform a demographic and psychographic study on your 20 percent. The better you get to know them, the better you can target them. This is where zero-party data comes in.
Zero-party data is data that is intentionally and proactively shared directly by the consumer, which you can use to determine what your customers intend to do or buy in the future. By collecting this data you will receive details about your audience that will help you better understand them and their needs on a personal level. By extension, you can use this knowledge (meaning data) towards personalized campaigns that are based on their preferences. Zero-party data will help your brand get a better grasp of the LTV of your different customers, thereby helping you make strides in acquiring additional customers that demonstrate similar LTV, especially when using a LTV predictive model.

You can read more about how zero-party data helps break new grounds in one of our previous blog posts. As you go through the post, you will see that the data science element of all this has a way of bringing everything together in a way that’s pretty much guaranteed to work.
How to think like a data scientist
At the risk of sounding like a total geek, there’s something fascinating that comes from delving into the 80/20 rule through the eyes of a data scientist. That’s because the deeper you get into it, you come to realize that the data is telling you a story. And not just any story—an actionable one!
I’ll use one of our own case studies as an example, so you can see what I mean.
This customer is a major B2C brand. Our analysis and demonstration of the Pareto principle will be based on their actual observed numbers and statistics.
Overall, we’re looking at a rather large population (their set users) consisting of roughly 3.5 million users. These users have all been acquired at least several months ago, which allows us to look back on the value produced by each over time.
We saw straight away that out of all of their massive population of users, only about 10 percent produced any revenue. 100 percent of revenue came from 10 percent of the users.
This is of course known, and the very purpose of marketing is to acquire the users who are expected to generate revenue. However, within those who do, the value is not distributed evenly. With that in mind, the rest of our analysis will focus on the paying users only: the 10 percent slice of the population.
By zeroing in on that 10 percent, we can sort the users by their LTV and plot the cumulative distribution.
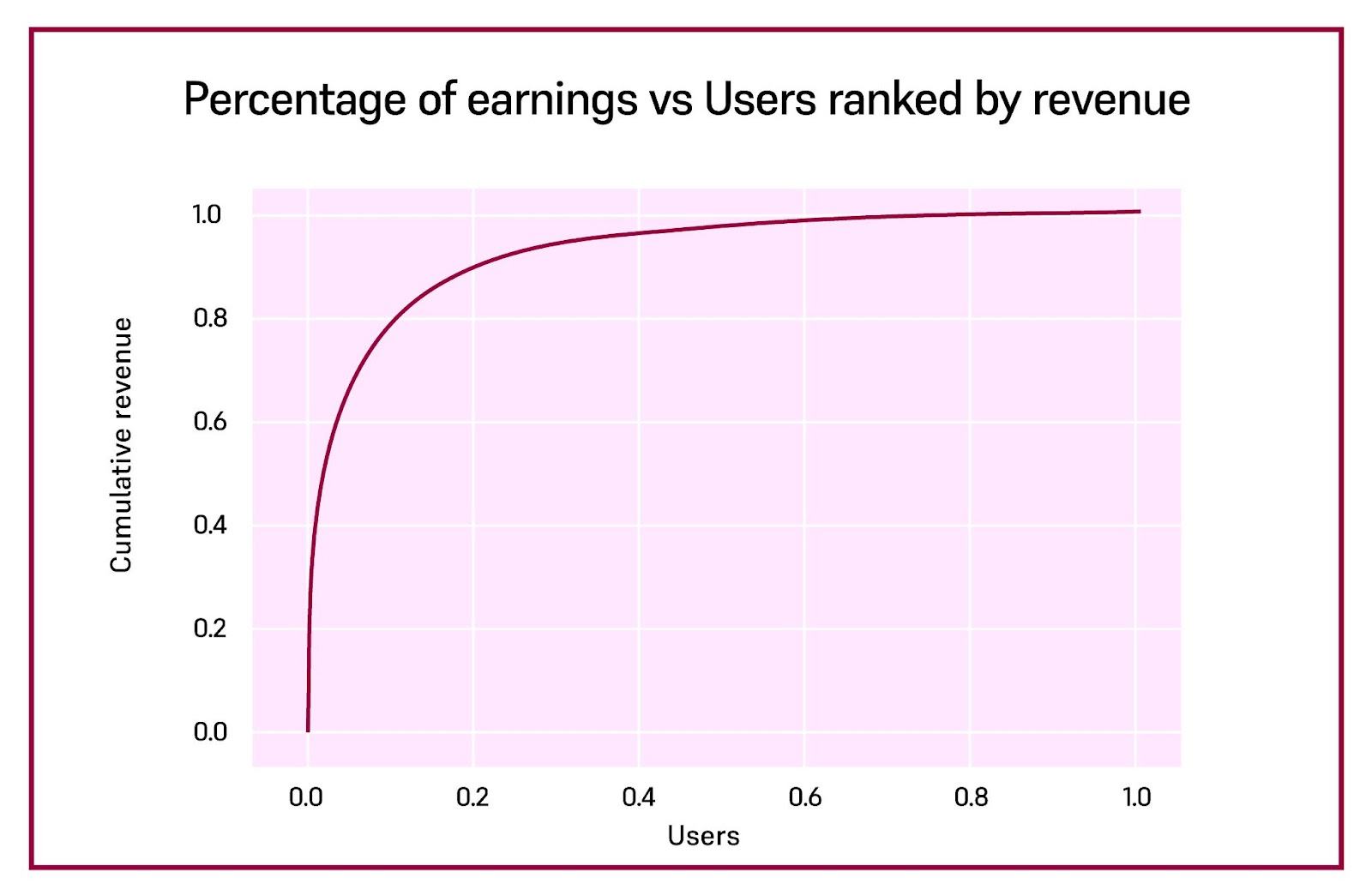
As you can see from the figure above, a very large portion of the total revenue is produced by a fraction of the users who produce any revenue at all. Out of the paying users, 20 percent account for ~90 percent of the revenue. ~10 percent account for ~80 percent of the revenue.
That is one percent of the overall population! Clearly, targeting such users can be of significant benefit.
Factoring data on the distribution of time
Now that we’re clear on how to analyse the distribution of value over users. We will now look at the distribution of time.
When doing this, it is interesting to ask: overall, does most of the revenue accumulate in the first few days since the acquisition time of a user? Or does users’ payment spread out evenly over time (or otherwise)?
To answer this question, we record (for each user) the day they made their first purchase since the time of acquisition. Below, you will see that we plotted the cumulative probability of paying within the first 1,2,3, etc. days.

We see is that overall, if a user has made any payment at all, it is far more likely that it will occur in the first few days since acquisition.
You can see that 90 percent of the first payments occurred within the first 50 days. By day 200 virtually all first payments have been made (except a negligible few), 90% occur within the first 25 percent days (out of the 200). So it’s safe to say that users tend to make their first payment rather early, or not at all.
But hmmm wait. Does this mean that the users who pay early are worth more to the brand?
To find out, we can look at the fraction of total value produced by all users as a function of the day since acquisition.

In the chart above, you can see that users who pay early tend to pay dramatically more than those who do not.
However, we can also see that this distribution is long-tailed. After 200 days, users continue to produce value, albeit in much smaller increments w.r.t the one produced in the first days.
Furthermore, it is useful to note that we’re playing the long game: a few large payments are nice to have, but we’re interested in the lifetime value of users.
The cumulative value vs the day since acquisition is shown below. This shows the fraction of accumulated revenue per user vs the day the user was acquired, averaged over all users.
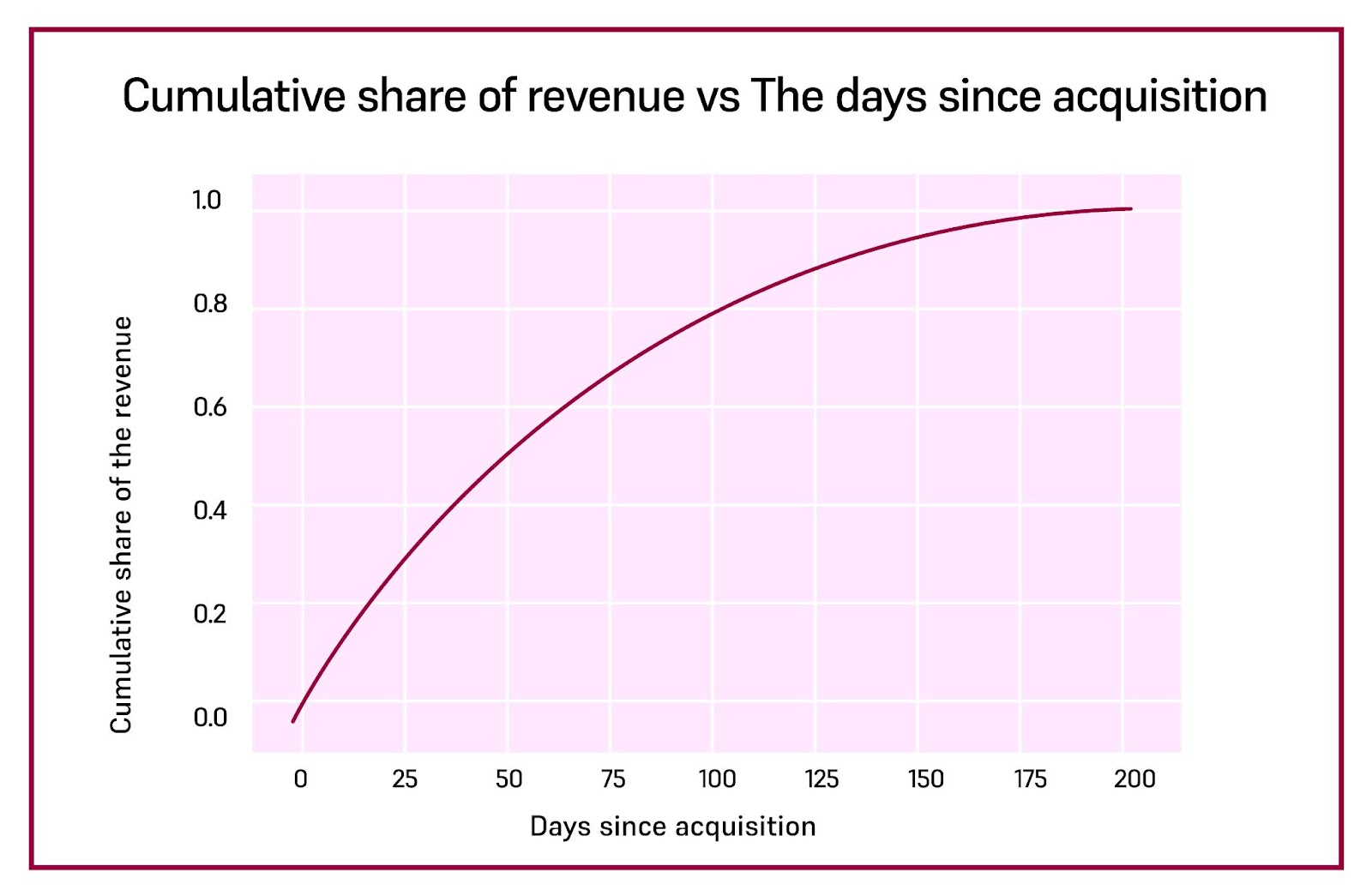
In this case, you can see there is less of a Pareto principle. The value continues to accumulate even after 150 days, that is, five months after acquisition. So it is well worth it to invest in finding users who do not make all of their payments necessarily early on.
In fact, we can make an explicit dichotomy between the “early birds”, who make any payment within the first seven days after acquisition, and “late-bloomers”, who make their first payment after the first seven days.
Of all of the paying users, ~55 percent are early-birds, and the rest, nearly a third, are late-bloomers.
Is that a bad thing in this case? Not necessarily. Theoretically, it’s possible for the early-birds to be worth much more than the late-bloomers, in which case there’s no need to bother with the latter.
Looking at the total revenue produced by all early-birds, we see they produced ~68 percent of all of the revenue.
Specifically, this means that targeting users who are expected to pay early can potentially make us miss as much as one third of the total revenue. So, for example, if an ad-network strives to target more users similar to those who were previously observed to be early-birds, it may end up missing a large chunk of the late bloomers.
Additional ways this shift can dramatically improve your marketing strategy
As you can see, when you consider the Pareto principle as a starting point for your data analysis, it’s kind of like going into a YouTube rabbit hole—endless good data. 🏆🏆🏆
Data science helps identify opportunities, and the sky's the limit when data science is leveraged for predictive models. That will empower you and your team to act, and acquire more users like your top 20 percent that demonstrate highest LTV, and a few other benefits and opportunities will have a way of also springing up.
For instance, less time/money/resources will be used when you know exactly what to hyper-focus on. Efficiency increases significantly with predictive LTV-based marketing. As a result, there will be an increase in productivity, better processes, and more ROAS/profits. Oh and one more thing—the data collected can benefit other departments as well, such as the product team and customer support. WIN!
Since we are collectively navigating through the new normal, now would actually be the best time for you and your brand to take the first step in making this shift. Greater scalability and profitability awaits!
Subscribe for more
Read expert stories, interviews, reports, insights and tips for profitable growth.