.webp)
Predictive LTV UA Optimization — What Does it Mean, and Why Will It Soon Not Be Just a Big-Brand Game?
What if you could freeze time just as a user is about to be acquired, and have all the time in the world to predict their behavior based on your historical data? Then you could decide which users to target, at what price, and what returns you could expect at any given time.
This imaginary future is somehow already happening, at least for the biggest online and mobile brands. It’s gaining traction and becoming the standard, by running user-level LTV based predictions and optimizing UA campaigns in accordance.
LTV based optimization is what makes acquiring VALUABLE users AT SCALE feasible. Developing it internally is sort of like rocket science (or is it quantum computing these days?), but as demand grows, end-to-end external solutions emerge.
We are all slaves to ROAS
In essence, ROAS answers the fundamental marketing questions such as “What yield would I eventually get for investing X dollars into this marketing channel?”.
This measure, looking at the long term, is much more accurate than, say, the return by a conversion window of 7 Days. Measuring and optimizing on short-term results is easier, and safer. However, the real prize lies in managing and hedging the risks of longer-term returns.
What ROAS should you aim for? It really depends on the industry, based on what I’ve witnessed working with brands in various industries. For instance, you should aim for a 20% ROAS by the first month if you are a SaaS company. Casual gaming is 2–10% from IAP. Hyper casual gaming it’s more about 20–50% in the first week. eCommerce aims at 70–95% within the first month, etc.
** Predicting LTV can be done at different levels and in different ways (e.g., cohort vs. single user). This topic merits its own blog post, which I promise you will get.
User-level LTV-prediction is like mining diamonds — Hard, but totally worth it
We are about to venture into the land of giants…
Nowadays, such advanced capabilities are mostly developed internally by top brands, using heavy (if not enormous) engineering and data science.
Soon, such heavy lifting will no longer be a necessity.
Most big brands no longer rely on the traditional go-to strategy of simply optimizing on cohort-level predictions and making a keep/kill decision. Neither do they settle for optimizing on the low-hanging fruit of short-term conversion windows that reach only a part of their target audience.
Utilizing their own data lakes, here is their methodology: First they lean on their own internal user-level data (user profiles, purchase behavior, engagement patterns, and multiple 3rd party data, to name a few) and run pattern recognition to identify patterns that segment their users into buckets of long-term value and retention. Then, with some mathematics, statistical methodology, artificial intelligence, machine learning, and other stuff smart people know, they build accurate and valuable LTV-prediction models. Of course, richer raw data yields more accurate models.
Every new user, therefore, receives a score that represents a prediction. You can forecast to what extent they will be profitable. Since users are also tagged by the campaign, ad group, or ad that brought them to you, you can learn which of your campaigns are ‘LTV better’ than others.
Up until recently, the way to act on this ability would be to make keep or kill decisions, divert budget and adjust bidding strategies for campaigns, ad sets, creatives and keywords, every few hours or days. This is achieved by aggregating cohort-level predictions on pROAS (predicted ROAS). This, by itself, was something only big-brands achieved, and it gave (and still gives) them an amazing competitive advantage. However, there was no way to leverage the user-level prediction, and bid differently for users in different value buckets. There used to be no way to automate this without explicitly building a real-time bidder (a huge undertaking usually facilitated by 3rd parties such as AppNexus etc).
Today, LTV based optimization is no longer a matter of keep or kill campaigns or ads. The new Facebook conversions API and Google’s Server-Side Tagging allow media buyers the integration that is essential to fire back server side signals, such as LTV, in order to optimize campaigns based on them (to be more specific and quite technical, it allows brands to share user-level signals, server to server, directly and not through the client endpoint device. Without it, sending an LTV score for optimization purposes is simply not possible). This new technology allows brands to send all sorts of new signals — offline conversions, time passed from a free trial, etc…The more sophisticated brands can use it to send LTV.
Diamonds for the masses
The above makes it pretty easy to understand why only a few iconic brands managed to build such monstrous machines in house.
But things have changed, technology has developed and demand pushed new types of end-to-end solutions that require zero R&D — Voyantis, for example.
Interestingly, Criteo says that 69% of organizations monitor LTV, but they do it inefficiently. Out of the ones that managed to do a good job, 81% improved their performance.
When asked, these were the barriers cited for proactively managing and monitoring LTV: too expensive, do not have in-house skills, too complicated.
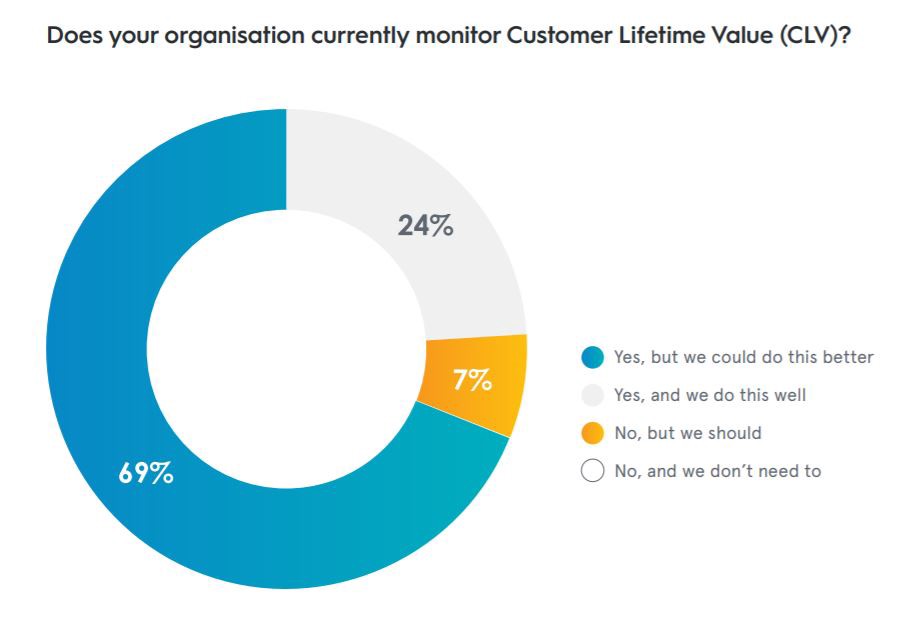
A case in point
A relevant example of a company, that I think did a really good job of measuring LTV in a way that can be acted upon,is Lyft. Lyft’s growth strategy focused on continuous improvement to the user-acquisition process, with a data-driven cross-functional methodology that considers scale, measurability, and predictability. For this purpose they developed what they call a ‘symphony architecture’, comprised of three parts: lifetime value (LTV) forecaster, budget allocator, and bidders. Read exactly how they did it here.
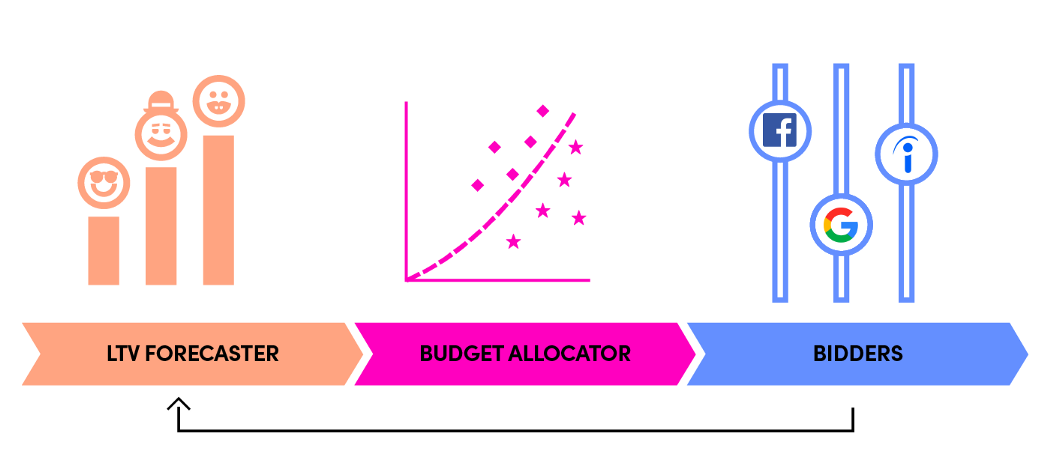
I believe that brands, and not just the biggest ones, should leverage AI to optimize marketing and growth activities. I know many growth professionals that are proactively searching for feasible solutions.
Currently, too many brands lack resources and expertise. This is exactly what my partner and I had in mind when we decided to found Voyantis.
Subscribe for more
Read expert stories, interviews, reports, insights and tips for profitable growth.